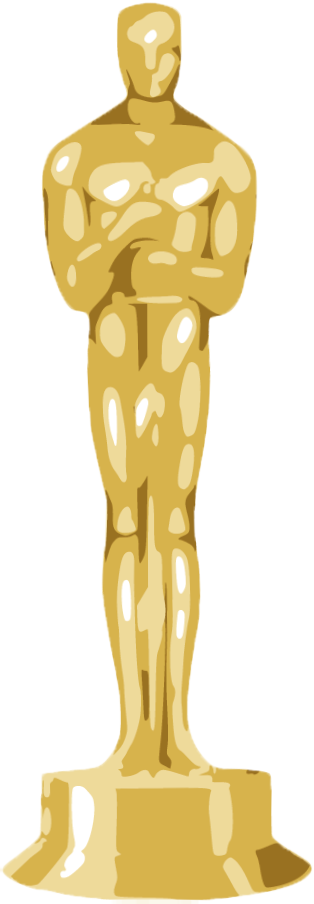
The Oscars. That golden little statue everyone is talking about. Answer me one question: wouldn’t it be cool to be on the red carpet, meet all the famous and infamous artists from Hollywood and feel like a star for one day?
In this tutorial, I am going to show you how to win the VFX Oscar. And we are going to do it without any third party plugins!
Let’s begin!
Step 1: Become a VFX Supe
God Bless America (or at least its supes)
Although over the last years we’ve started to see more diversity, American supervisors are still a huge majority amongst winners and nominees. Go to America and get the American citizenship (if no wall in the border prevents you from doing so).
As you can already see, this is going to be easy-peasy.
Choose your birthday wisely
Could your birthday influence your career in any way? Well, spoiler alert, it pretty much does.
But how? In 1985, a Canadian sociologist realised that all the players from the Canadian national hockey team were born in the first half of the year. He later found that most players in sports teams were born in the first 6 months of the year. It’s called “Relative Age Effect”. Google it.
In the USA, we find that more nominees are born between September and January (the academic year in the USA starts in September). For the rest of the word, the academic year starts in January and, as we expected, we find the majority of nominees from January to June.
If you do not meet this criterion, consider being born again in a different month.
Be mature, but not old
The optimal age for winning an Oscar is between 35 and 45 years old. The optimal experience in film is between 10 and 20 years.
This means that the majority of the winners start at a very young age. This trend has been accentuating over the last years. In the 80s, supervisors started in VFX at the age of 29. On the last 10 years, this has gone down to 25 and a half.
Children, stop playing Pokemon Go and start doing rotos.
Be male, straight and white
Last year, Sara Bennett became the first female supervisor to win the Academy Award for best visual effects. Before her, there had been only 1 woman to win the award and another nominee.
So technically, there are almost as many women as there are in the Best Actor category.
We can do better.
Step 2: Make a movie
Spaceships blow up superheroes and dragons
Make sure your movie is a sci-fi. Over 46% of the movies that have won an Oscar are sci-fi. And even tough we all want to be batman, superhero movies will drastically lower your chances of getting the golden trophy.
Sequels and franchises are not welcome to the Oscars. The more movies have been done before with the same theme, the more the probability will drop.
3D dinosaurs are a thing of the past. Nowadays having an organic CG creature won’t make that much of a difference. It will if it plays an important role in the story. It is all about the story.
But most important: practise rocket science. Be innovative, develop new tools and make them public. Doing so doubles your odds of becoming the winner. Take as an example deep compositing or a new motion capture system. Non-technical achievements such as having a nominated female supervisor also count as VFX Milestone.
To sum up, team Vader beats team Batman and team Potter.
Remember remember the 5th of December
Once you’ve finished your movie, wait to release it until July or December. Almost half of the movies released in these months won the Academy Award.
Strangely, over the last 25 years, no movie has ever been released in January, February or September. This year’s Kubo and the Two Strings, as well as Deepwater Horizon, have both been released in September.
Also, make sure to make your movie profitable ($ earnt vs. $ spent). Awarded movies were +37% more rentable than their competitors.
Seduce the critics
Now pay attention. Your Oscar may depend on this. This is the key, the secret ingredient, the ultimate answer to life the universe and everything.
You need to make a good movie. One the critics will fall in love with. One that will get as many nominations as possible in the Academy Awards. And here’s why: the VFX Oscar is (almost) always for the best movie.
Look at the three graphs right here. Every dot is a movie, and they are higher or lower depending on their IMDB score, their Rotten Tomatoes score or the number of different nominations they got in the Oscars. Light blue dots represent nominees, and the darker slightly bigger ones represent winners. It may look random at first, but look closer. If you look at each year, each column, the top dot is almost always a winner.
Odds are a movie has a 30% chance to win AND be the movie with the highest score. But in practice, this happens around 80% of the times.
If you want to guess who is going to win the Oscar, count the number of nominations of each movie and pick the highest one. You can be almost certain you’ll get it right.
Just let that sink in.
Step 3: Guessing the winner
Building the brains
Now that we have all of this data, could we use it to guess the following year’s winner? Some people have faced this problem before, but how could we raise the bar? If only there was a way to teach a computer how to predict the awards! But guess what? It exists and it’s called neural networks.
We can build a network which takes all the information about the movie and returns a number between 0 and 1, based on how strong the movie is. Then, for each year, we take the nominee with the highest mark, and crown it as the winner.
But the Oscars are not decided by a single individual, but instead, a group of members get one vote each. In a similar manner, we will have 51 different neural networks, each with its own different opinion.
Finally, we will let the network train by trying to guess all the previous year’s winners. After 1 week of training, it had a 94.9% accuracy.
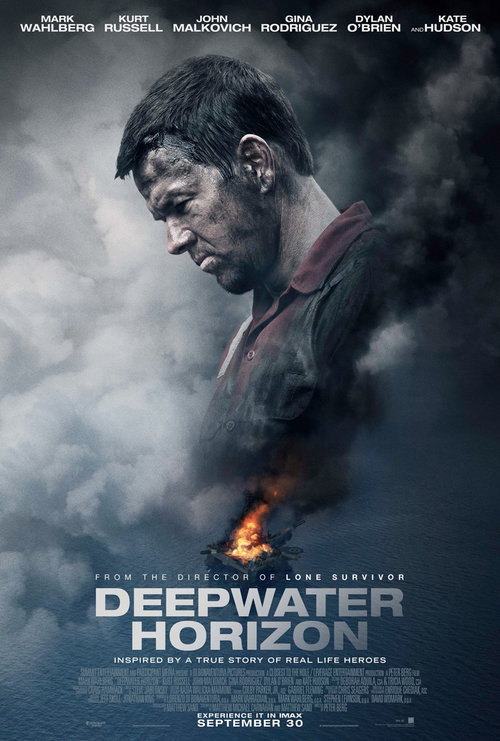
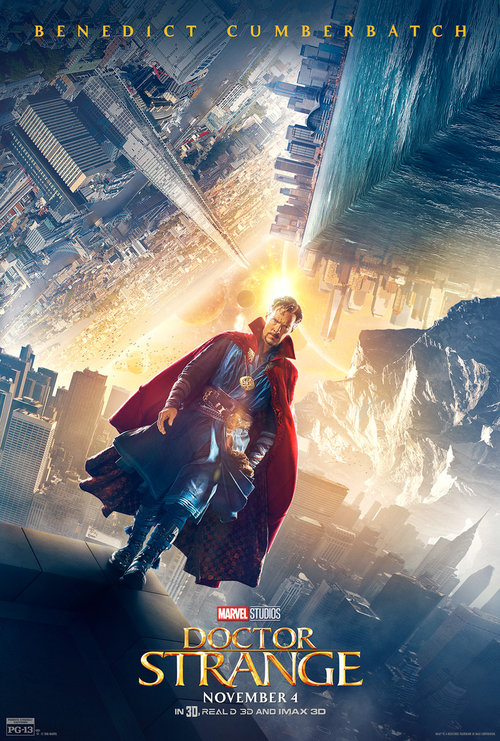
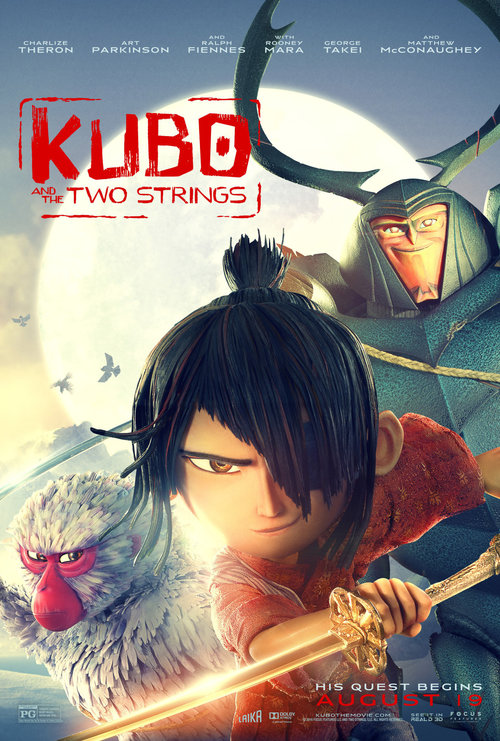
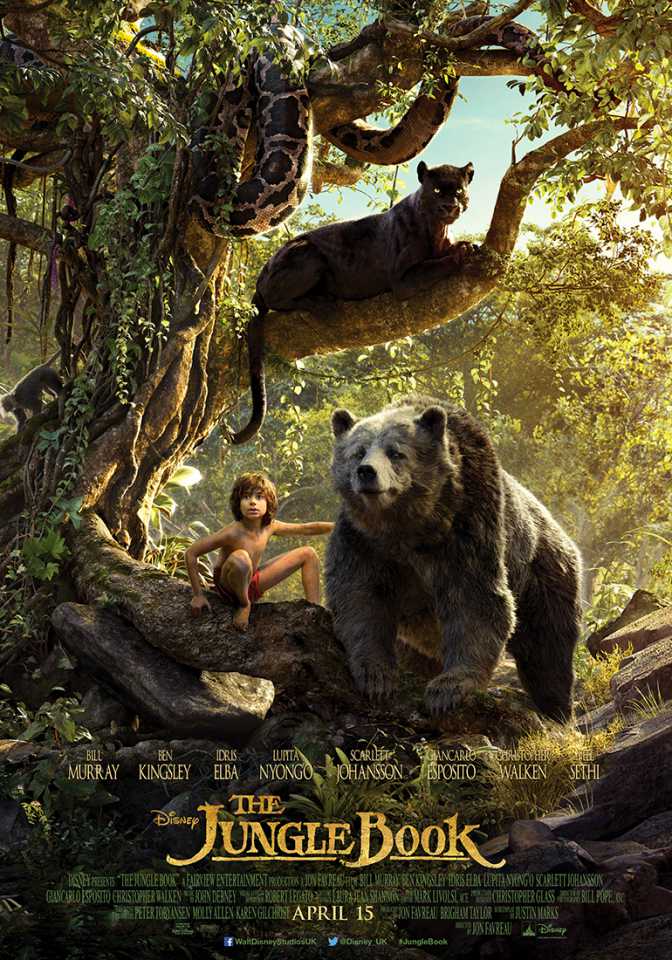
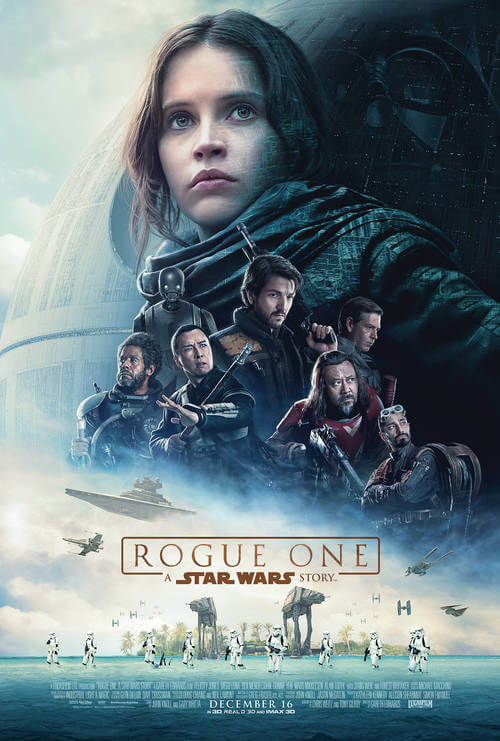
This 5 movies are competing for the VFX Oscar.
But who will win the golden statue?
And the 2017 winner is…
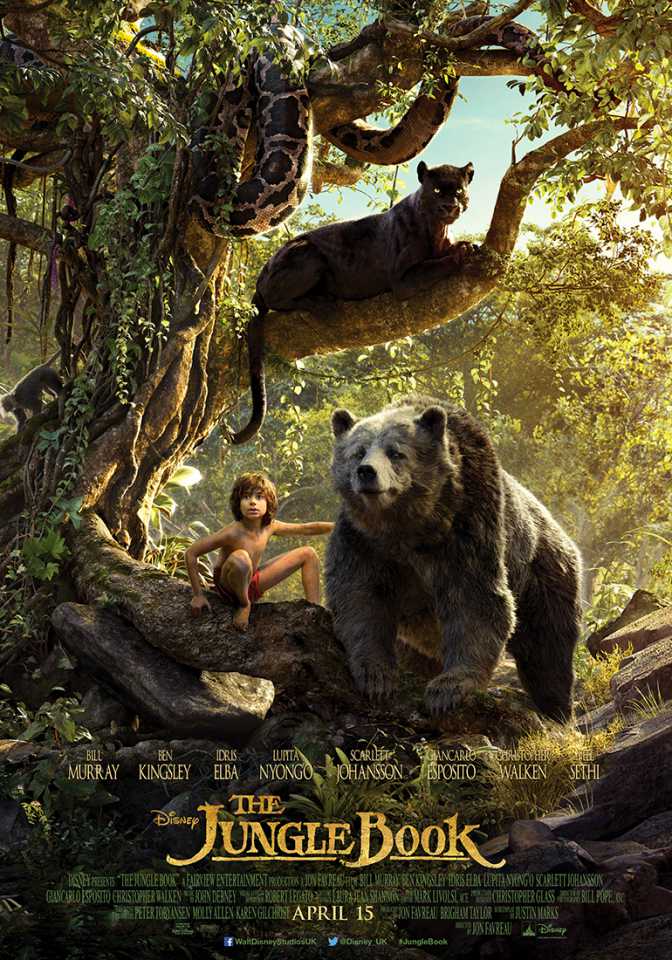
With just over 50% of the votes, The Jungle Book rises strongly against its competitors. The huge number of photoreal animals that appear in the movie, the experienced director and supervisors and it’s amazing scores in IMDB and Rotten Tomatoes give all the odds to the man cub movie.
But keep an eye on Kubo and the Two Strings. After last year’s Ex Machina, Kubo may be the perfect surprise for the night.
Other fancy graphs
Supervisor data
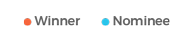
Movie data
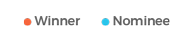
A short note from the author
First of all, I am an FX TD, not a statistician. I’ve written this article out of a boring Sunday. This is not a scientific paper nor a professional study, so please, do not take it seriously. My primary sources have been IMDB, Wikipedia, Rotten Tomatoes and FilmAffinity. For the supervisors’ names, I had to do some further digging, in some cases, I even asked them via twitter (and some of them replied!).
I looked at all the movies from 1977 to 2016. For the supervisors, I looked between 1990 and 2016 (458 people in total!). I couldn’t get everyone’s nationality nor birthday. In total, I got 80% of the nationalities, 59% of the ages and only 43% of the birthday’s months. I want to insist in the fact that these datasets are incomplete.
For the deep learning, I used inputs such as box office, budget, the experience of the supervisors, if it is a part of a sequel, total nominations to the Oscars, between others. I did not take which VFX studio had made the movie in consideration.
When running the deep learning network, I would train it with incomplete sets of data, in order to validate the learning and avoid overfitting. Ex Machina seems to be the main reason why Kubo has such a great potential for the Oscars. If I hadn’t given Ex Machina a “low importance”, Kubo would win with over 60% of the votes. I don’t want to say Ex Machina didn’t deserve the Oscar, but for sure it didn’t follow the statistical patterns I was trying to teach the neural network.
And last but not least: correlation does not imply causation.
Ok, that wasn’t that short…
“The complexities of cause and effect defy analysis.”
Douglas Adams